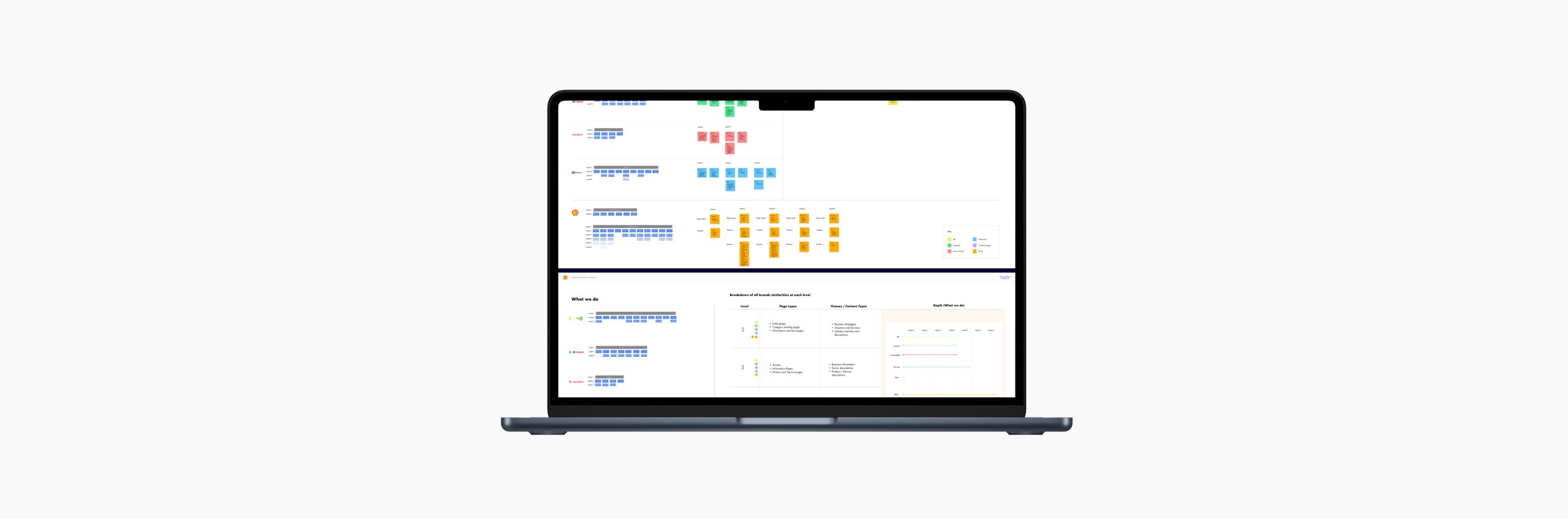
Shell IA & navigation
Background
The Shell global website is overcrowded with duplicated content, has limited content hierarchy, uses complex business jargon, has poor navigation, contains four times as much content as its competitors, and lacks a human touch.
The website provides a passive experience rather than proactively driving user actions. It also lacks clear directions to guide customers through their experience.
Challenge
The website serves various user groups, each with specific goals and information needs. The current information architecture and navigation make it difficult for users to find the information they need, leading to high drop-off rates and causing users to turn to competitor sites.
MY ROLE
Senior UX Designer
Competitor analysis | User persona’s | AEM data | Card sorting | Tree testing | One-to-one interviews | Scoping | Candidate recruitment
THE CLIENT
Shell Global
TOOLS
Figma | Maze | Excel | PowerPoint
Competitor research
The Shell website is a complex mind field. By analyzing the information architecture (IA) of Shell and its competitors, we were able to identify similarities and differences that would have been difficult to find otherwise. We found that Shell’s IA surpasses that of its competitors in the first three levels and extends even further by another three levels. This wasn’t at all a surprising insight.
Additional top-level inconsistencies that were identified were:
- Varying article structures
- Inconsistent landing pages
- Articles categorised incorrectly
- Dissimilar naming between URL, menu title, breadcrumbs, and page headings
- Floating/uncategorised pages
Key findings
- Shell in regards to the competitors that we researched was the anomaly when it came to the IA and the terminology. There was an array of similarities with the competitors. Shell had chosen their own business jargon and expected their customer to learn in order to navigate the site and find content successfully.
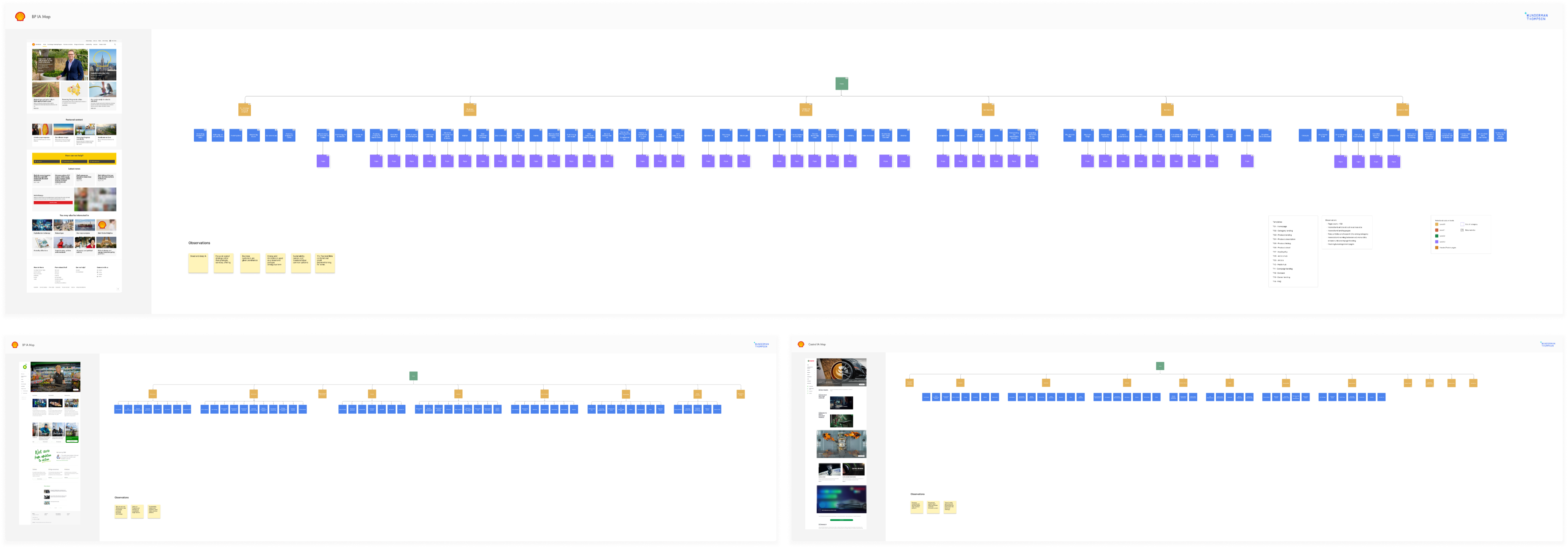
Shell and competitor IA structure
Visuals of IA’s were a great starting point for this project, allowing us to begin identifying correlations and disparities.
The terminology used within the navigation was a further major issue for users to understand where content was to be stored within the site and what specific business jargon meant. Mapping out levels 1-2 of the navigation would allow us to highlight terminology similarities, navigation header, content groups and labels.
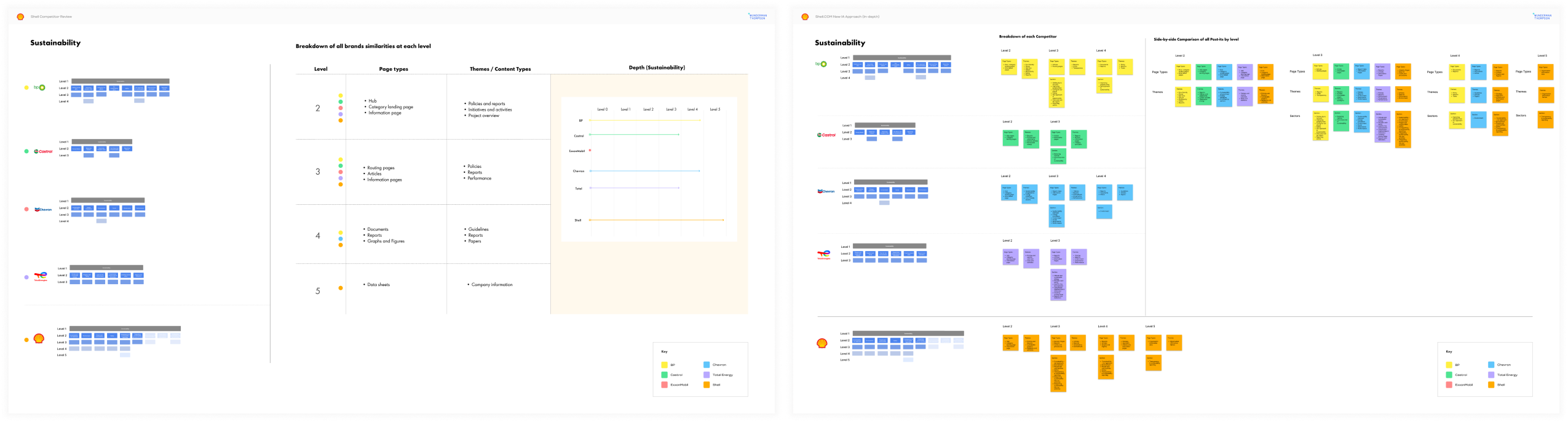
Shell and competitor terminologies
Persona development
Working alongside the Shell data team, we identified the user groups to focus on: Investors, Journalists, Rating agents, Energy consumers, B2B customers, B2C customers, Job seekers, and Shell employees as these were the main drivers of the website.
Utilising AEM (Adobe Experience Manager) to gain a deeper understanding of the 8 provided personas, we spent the next couple of days analysing the data to identify persona-specific journey patterns.
These data-backed personas would be the entire backbone of the project and the key for success. By keeping the needs and expectations of these personas front of mind, we could ensure that the future IA & nav would effectively meet their requirements.
Data questions
- What were the entry and drop-off points where
- How long were they on the site
- What category in the nav did they interact with
- Common pattern journeys
- Type of device
- What were they searching for
- Were there correlations between their first click on the nav and where they ended up
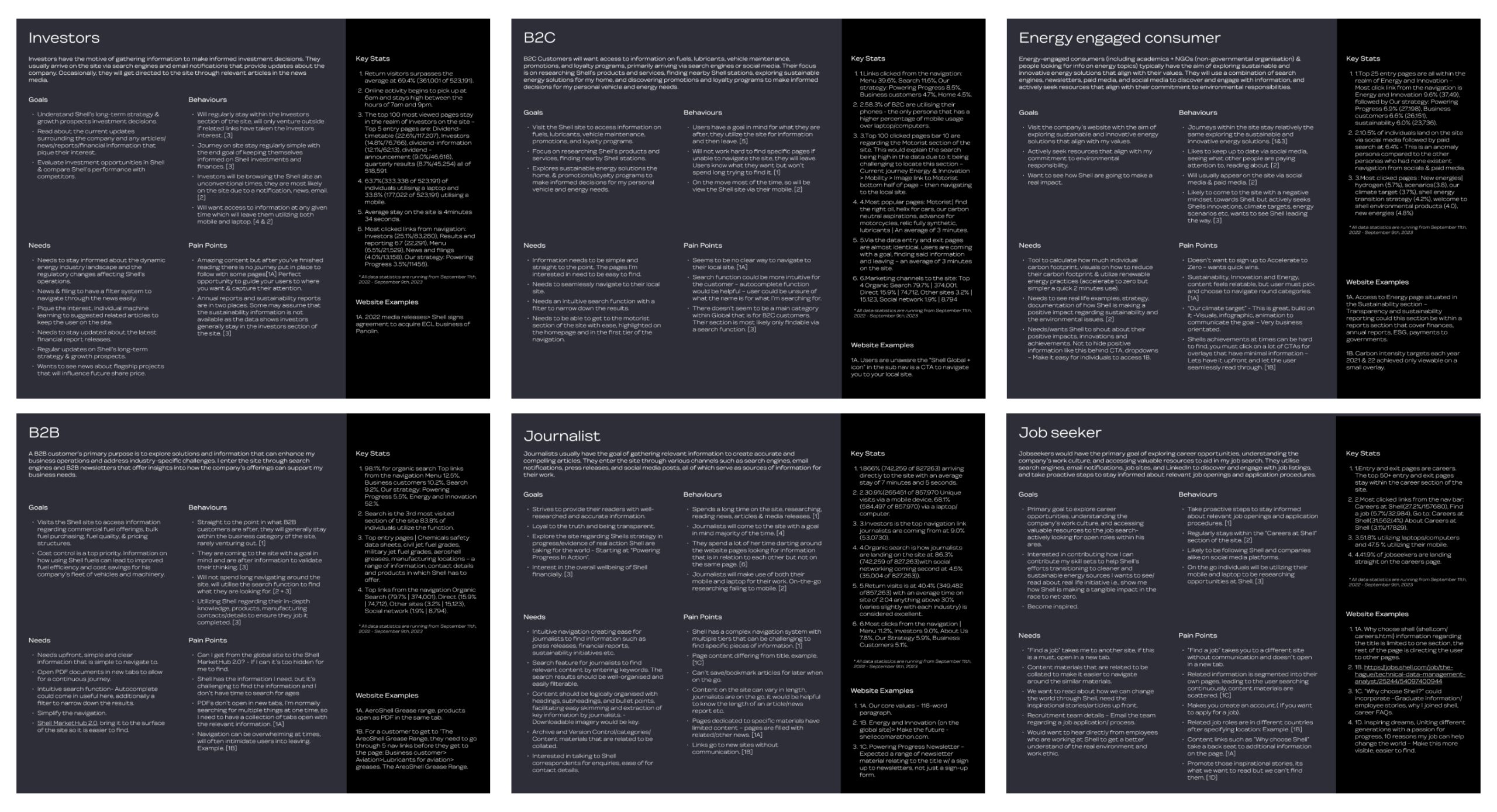
Data backed persona’s
Card sorting
Never a dull moment when it comes to user testing, especially when you are testing 100+ people for a card sorting exercise. Terminology and content groups were vital parts that we needed to get right and who best to lead us to the right answer than the users themselves.
The data-backed personas allowed us to obtain a comprehensive understanding of our eight key personas, allowing us to be selective when recruiting participants. We conducted a one-to-one interview with one participant from each persona, while the remaining participants completed the card-sorting exercise independently.
Our approach
We took a hybrid approach to the card-sorting task, we wanted to understand how our users would group the terminologies together and under what header. Utilising Maze we brought our test to life with a range of questions about the participant and their knowledge towards Shell.
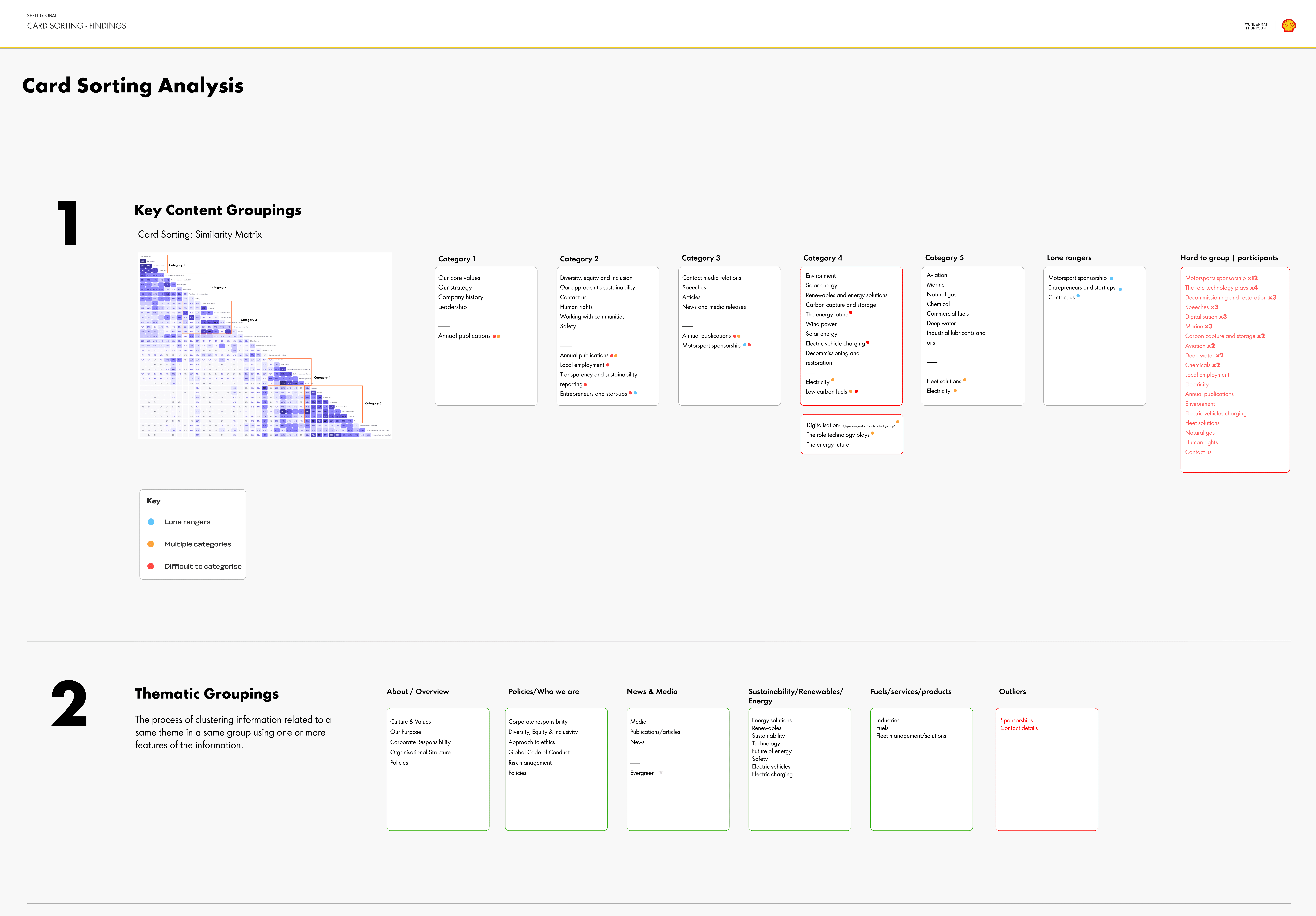
Data analysis from card sorting
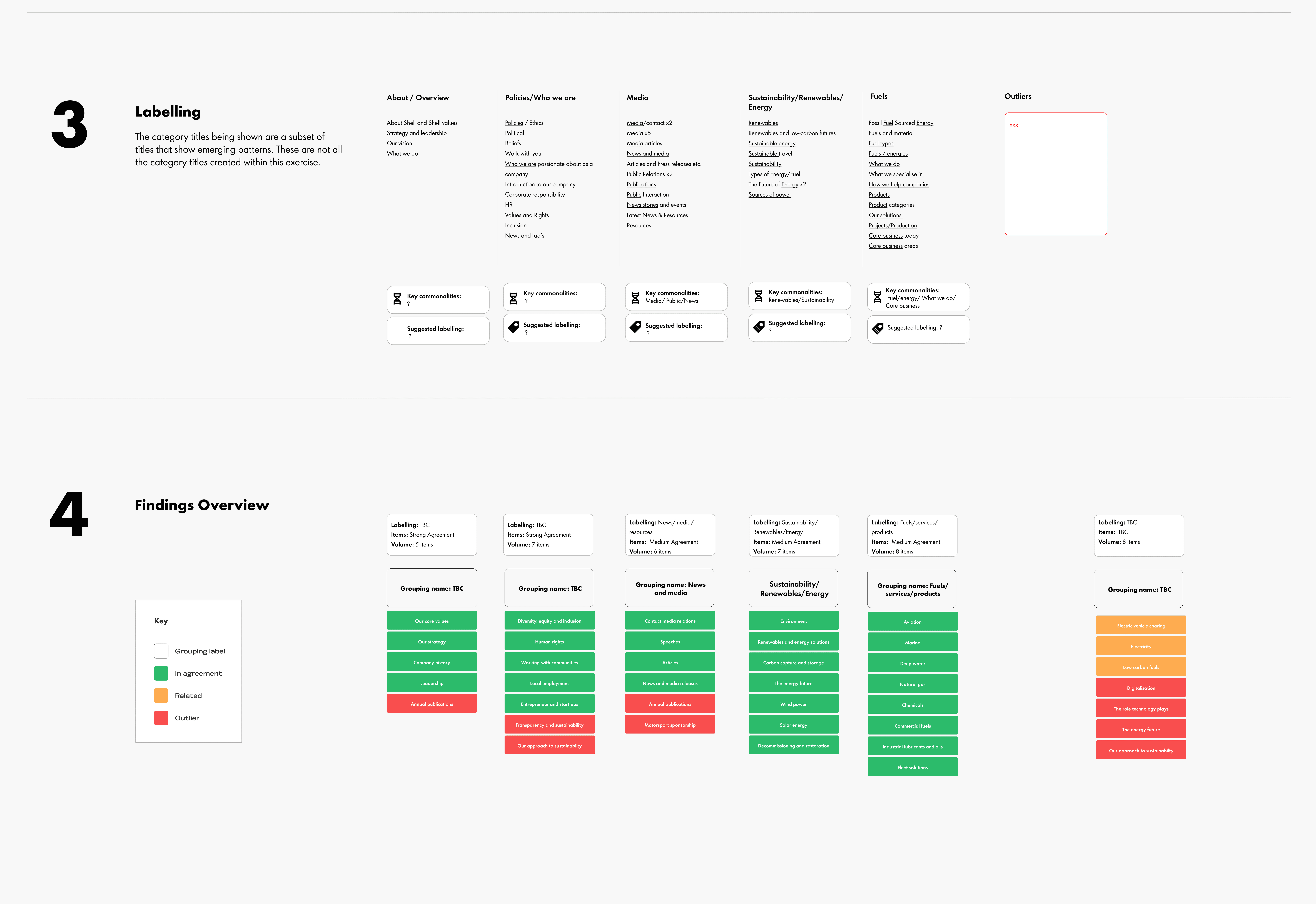
The data we received back from the test was overwhelming, to say the least, but incredibly valuable to the success of this project.
Understanding how our user groups are thinking when it comes to the navigation, and what terminologies mean to them compared to what they mean to the business was so intriguing. There were a couple of disparities that needed further investigation before we could make final decision for the site which lead us to a second user test this time being a tree test.
Insights
Studying and questioning our selected consumer whilst they group terminologies allowed us to peak and disect their mindset. Gaining a wider perspective as to why they would categorise in a specific way whilst highlighting what terminologies didn’t make sense to them.
We quickly noticed a pattern: our consumers would instinctively choose the easiest and most straightforward terminologies to group, leaving the controversial or hard-to-group terminologies for last. Recognising this pattern allowed us to adjust our testing strategy to ask the consumers more specific questions about these terms.
Tree test
The data from the card sorting test was invaluable but there were still a few disparities. To obtain a more comprehensive understanding of participants’ preferences, two tree tests we conducted to compare various labelling variants and pathway structures.
The purpose of these tests was to identify the labelling variety and pathway structures participants most favour. The test results would enable us to develop an improved IA that is tailored to meet the customer needs.
Data collected from the tests would be analysed to identify patterns or trends that could inform our decision-making process. The tree tests facilitated an informed decision about the labelling variant and pathway structures to be utilised.
Insights we are looking at:
- 12 tree test questions to go through with the most controversial insight at the beginning
- Insights into tasks or areas the participants found to be particularly difficult
- Insights into where participants would expect to find Motorsport Sponsorship content
- Insights into where participants would expect to find an overview of the company’s major projects
- Insights into what sort of topics participants would expect to be covered in a section called “Mobility”
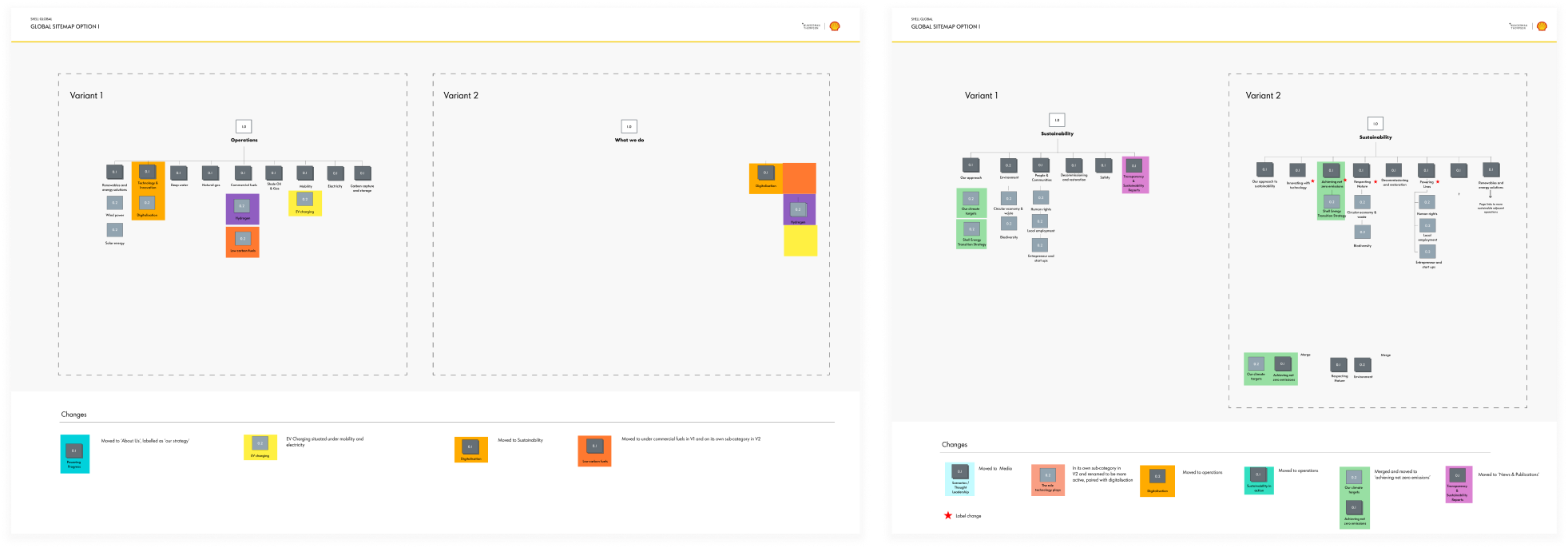
Tree test visual (figma)
Next steps
Once we had completed the IA & nav work and the client was happy with all the changes and recommendations we would work with the Shell developers for the next could of months to ensure everything we had recommended was implemented correctly .